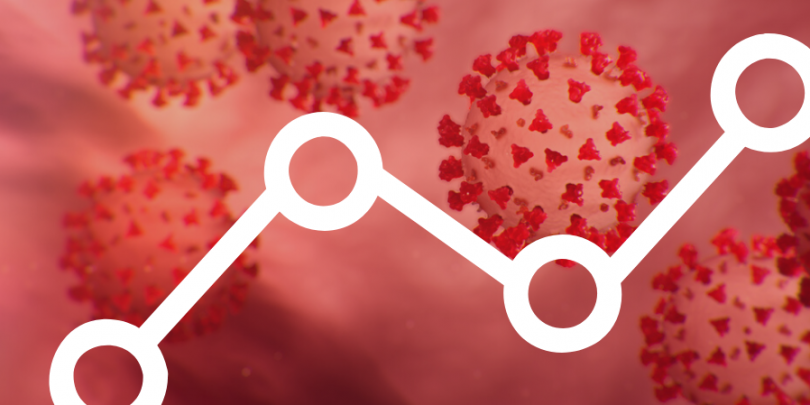
Using Data Analytics to Make Informed Decisions in the Time of COVID-19
How can we leverage data analytics to help us make informed decisions during this COVID-19 crisis? Access to real-time data on COVID-19 cases can help us determine potential hotspots, allocate resources (e.g., test kits, masks, and other protective gear) to those areas and prevent the spread of the virus to other locations. But we should always be mindful of the limitations of data, and what is hidden from it. What is the data not telling us?
I am a business school professor, not an epidemiologist. Although I may know very little about epidemiology, I use data analytics in my research1,2,3,4,5,6. In my experience of working with large amounts of data, I have learned that unlocking useful information from the data is not easy. Data are messy. (Yes, it is plural.) There are many limitations to the data. The real challenge is on how to leverage our observations to make better decisions. Our goal is to translate the data into something prescriptive.
There are many examples of how data analytics are helping us make informed decisions during this crisis. For instance, a team of scientists and doctors used data on the number of COVID-19 cases in Wuhan from December 31, 2019, to January 28, 2020, to infer the actual number of infections from December 1, 2019, to January 25, 2020 [7]. By doing so, they were able to calculate how fast the virus may spread to other cities in China and globally.
One of the most successful countries in managing the spread of the disease is South Korea [8]. The government used data to create a map of where the infection was; this informed citizens whether they were likely exposed to the virus or not [9]. Their aggressive testing protocols had direct and indirect benefits. First, they were able to capture how fast and where the virus was spreading. Second, this sent a strong global signal to limit travel to South Korea, preventing additional imports of the virus into South Korea [10]. Government agencies also used the data to target specific establishments that may have been exposed to infected persons. Instead of closing all establishments, they used data to identify hotspots [10]; their version of maximum quarantine is careful targeting as opposed to nationwide lockdown [10].
The state of Ohio was also fast to leverage data to inform its citizens of the spread of the virus. The Ohio Department of Health created a dashboard on the total number of COVID-19 cases by county [11]. (You can download the dataset in CSV format!) It shows the date of when each person was confirmed with the virus, their age range, county, and gender. These summary statistics can be insightful, but there is so much more information to unlock from the data.
We can use data to forecast resource needs [12], such as the number of respirators, ventilators, and other protective gear. Unfortunately, due to the incredible demand for protective equipment, there are not enough of them [13]. The capacity to produce them here in America is limited [14], but some global manufacturing facilities have responded flexibly and quickly [15]. In Operations Management, flexibility and speed are key competitive strategies. The ability of several facilities to shift swiftly from making traditional clothing to masks or from bottling alcoholic beverages to hand sanitizers is impressive [16]. However, if supply cannot rapidly meet demand, then the next best solution is to allocate the limited supply correctly.
We can use the data to allocate resources in areas where the disease is spreading faster than others [17, 18]. For example, we can merge the total count of COVID-19 cases by county [19, 20] to a dataset of healthcare providers across the US [21]. If the data are available, we can then estimate the capacity of each hospital or healthcare provider in the US. (Most hospitals have high utilization rates in general, but some will always be busier than others.) We can then identify areas where there is a high number of COVID-19 cases and facilities that have high utilization of protective gear. We can then prioritize the allocation of existing supplies based on levels of use. This is just one example of how data and analytics can help make informed decisions during this crisis. But identifying hotspots is not as straightforward as it sounds.
I always remind my students to be careful when working with data. It can be misleading if we do not scrutinize it. Let me illustrate this with a concrete example using the COVID-19 dataset. I downloaded the dataset of daily-recorded county-level COVID-19 cases in the US [19, 20]. The first recorded case in the dataset was on January 21, 2020 [20]. My purpose for downloading the data is to look at the progression of the disease across different states. The figure below shows the number of cases each day across US states and several territories. (I excluded New York, New Jersey, and the Northern Mariana Islands because the first two dwarfs the graphs and the last does not have enough observations.)
Notice how the curves all look different. We do notice similar patterns in states such as California, Massachusetts, Washington, and Wisconsin. We see a hockey-stick pattern, long and flat at the beginning, and sharply increasing towards the end of the panel. What factors do you think contribute to this variation? I also posted the same question on my Facebook, and I got an obvious response: test kits.
An important factor is the number of test kits available in each state. This might sound obvious, but this is an important observation. The data we see is the number of tested and recorded COVID-19 patients, but it doesn’t mean that every infected person is reflected in the dataset. This is what we call “censored data.” The data is not telling us the exact number of infected persons in the population. One possible reason for a hockey-stick observation is that there were a limited number of test kits in the early periods of the pandemic (but from my little understanding of epidemiology, infections do spread exponentially, so this is a natural shape to expect whether test kits were limited or not). The key takeaway here is that the observations we see are only a partial reflection of the actual number of infections, but some data is better than none.
Operations managers often work with censored data. Let me illustrate an example using demand, sales, and inventory. Suppose for a second that a store always maintains 100 units of toilet paper in stock and that they can instantaneously replenish this (a hard assumption to buy during this pandemic). The blue line reflects the inventory level in the graph below. Now suppose that the dashed curve represents daily demand. If the demand is below the inventory level, then observed sales is the same as demand. However, if the demand is above the inventory level, then we have a stock out. The maximum observed sales is equal to the inventory on-hand; it cannot be more than that. Therefore during stock-outs, our observation of sales does not fully reflect the demand for toilet paper. It is censored.
We can still make informed decisions with censored data, but we have to be aware of these (potentially) hidden observations. One approach to circumvent this issue is by developing mathematical models that require very few parameters to estimate what we would have observed in the absence of the constraints (e.g., maximum inventory of toilet papers or the total number of test kits available). We can apply this data-analytic approach to our COVID-19 scenario.
Operations Management (and in economics and statistics more broadly) is rich in methods in handling censored data [22, 23, 24]. We can use our knowledge of censored data to more precisely estimate the lack of resources (e.g., number of test kits needed). Even if we do not use sophisticated models that account for censoring, working with data can still help us prescribe informed solutions. We just have to be aware of the limitations of our observations and account for those when we make recommendations.
Collecting data is challenging but essential. Otherwise, how can we manage what we do not measure?