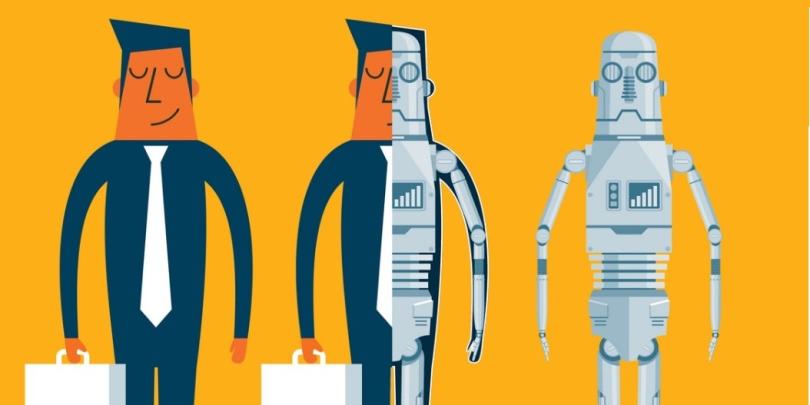
Is Artificial Intelligence Replacing HR Soon?
The development of artificial intelligence (AI) and how it is going to alter human labor is under furious debate.
Recently, IBM proudly announced they have developed a powerful tool that can predict employees’ turnover within six months of departure — all based on thousands of employee work records and AI. Without revealing the algorithms, IBM claimed the accuracy of prediction reached 95 percent [1].
After the press release, a concern arose that if the turnover predictive tool is 95 percent accurate, would artificial intelligence completely replace human resources employees and management teams? But here is my thought: AI is powerful in analyzing objectives and consistent data — but not interpersonal dynamics or unexpected conditions.
Why am I saying so? Allow me to share some research evidence with you.
- Turnover decision is often triggered by an unexpected event
IBM’s chief human resources officer, Diane Gherson, disclosed some factors the algorithms use, such as job tenure, pay grade comparisons (both internally and externally) and promotions record. These factors are not merely products of computational efforts and algorithms. In fact, the factors identified by AI were some that have long been studied.
For example, based on what is known as [2], (in short, this theory states people feel stressed and unhappy when their contributions to work do not match up to their compensation) if you have found yourself being continuously underpaid or not recognized in the current job, you would likely feel dissatisfied and take actions to reverse the situation, such as reducing effort in your current job. Searching for a new job would be another reasonable action plan.
Aligned with but not limited to the equity theory, turnover researchers identified employees’ desirability to change jobs and the ease of movement (including getting a new offer, the difficulty to relocate, etc.) as two core determinants of turnover [3]. The turnover-prediction tool identifies the risk factors that may lead to dissatisfaction —combined with an IBM-specific algorithm to enhance the predictability of turnover up to six months ahead of time.
However, later research further sophisticated the process and suggested that turnover actually needs a trigger – a shock to flame the decision [4]. The unfolding model proposes that, prior to having the desire to quit, an employee must experience an unexpected event – a shock to change his/her current life. A shock can be a mistreatment from the direct supervisor (negative), a new baby (neutral) or a promotion (positive). Even if the employee was sort of satisfied with the work, the shock stimulates the employee to think about the fit between his/her current job and his/her expectations of a job. If mismatched, the employee starts to feel dissatisfied with the current situation and begins searching for a new position. Once the employee finds a more appealing job, he/she quits.
Therefore, what if there is an unexpected event that overrides everything, such as when you feel you should have gotten a promotion but the the promotion instead was granted to your colleague? The predictive tool used in AI may be not work so well in such circumstances.
- Interpersonal interactions matter
Also, artificial intelligence can be powerful in identifying employees who care more about their paycheck and extrinsic success but not those who appreciate workplace relationships or life quality.
For example, positive work relationships can lead to a spillover effect of turnover. Researchers found that if employees have positive and mutually beneficial relationships with their supervisors, the employees are more likely to quit after their supervisors leave [5].
Moreover, leadership may lessen the detrimental effects of experiencing an extreme circumstance. An example would be an unexpected merger or environmental threat (hit by a hurricane or wildfire). Researchers surveyed 564 U.S. Army soldiers 12 months before their deployment to an active war zone overseas and followed up right after they were back. After 12 months of severe combat battles, research found that transformational leadership, which stresses collective vision along with individual needs and intellectual thinking, increases soldiers’ sense of embeddedness to the unit and decreases their intention to leave [6].
Therefore, leadership and leader-member interactions may cast more weight on determining turnover than the numbers on the paycheck or the algorithms.
To sum up, without accounting for problematic employees, the intention of developing such a computational tool should be to quickly diagnose workers’ needs so the company and supervisors can intervene to retain the employees.
What do you think? Leave a comment and share your thoughts with us.
References
[1] The new way your boss can tell if you’re about to quit your job (2019, April 11), The Washington Post. Retrieved from The new way your boss can tell if you're about to quit your job.
[2] Adams, J.S. (1963). Toward an Understanding of Iinequity. Journal of Abnormal Social Psychology, 67, 422-436.
[3] March, J., & Simon, H. (1958). Organizations. New York: Wiley.
[4] Lee, T. W., & Mitchell, T. R. (1994). An alternative approach: The unfolding model of voluntary employee turnover. Academy of Management Review, 19(1), 51-89.
[5] Ballinger, G. A., Lehman, D. W., & Schoorman, F. D. (2010). Leader–member exchange and turnover before and after succession events. Organizational Behavior and Human Decision Processes, 113(1), 25–36.
[6] Eberly, M. B., Bluhm, D. J., Guarana, C., Avolio, B. J., & Hannah, S. T. (2017). Staying after the storm: How transformational leadership relates to follower turnover intentions in extreme contexts. Journal of Vocational Behavior, 102, 72–85.
Disclaimer
Here at Lead Read Today, we endeavor to take an objective (rational, scientific) approach to analyzing leaders and leadership. All opinion pieces will be reviewed for appropriateness, and the opinions shared are solely of the author and not representative of The Ohio State University or any of its affiliates.